Beginning a blog with the dictionary definition of a word is the height of hackery, but I do believe that is actually the rudimentary level we need to stoop to in order to address the problem with Silicon Valley’s overhyped Artificial Intelligence and how it gets covered. Intelligence is defined as “displaying or characterized by quickness of understanding, sound thought, or good judgment.”
Let’s unpack that “good judgement” angle in the context of this so-called “intelligent” computer program.
This problem is not something that can be easily remedied either, as the foundational construct of A.I. is its dataset. Everything an A.I. “knows” begins and ends with the terabytes of training it has undergone. If you only fed it pictures of people where their hands were hidden, then asked it to draw pictures of humans, it would draw a bunch of people without hands.
Let me show you an example of this foundational logical flaw out in the wild, and the house of horrors OpenAI’s Sam Altman and his other PR flacks will have to navigate as they struggle to scale a technology that Sam Altman suggests needs $7 trillion in investment to truly work the way he claims it can.
Sorry folks, that’s got to be a typo there. Still getting up to speed on this whole Editor-in-Chief thing while finishing up school. No way he said $7 trillion. That’s just crazy talk. He said $7 billion right?
So we need to raise a little less than a third of the United States annual GDP, and then this technology that cannot understand really elemental stuff will magically figure out how to think for itself. This is sheer and utter madness. The hype has so far surpassed the current capability of this technology that when it does demonstrate real utility like the bird identifying app Merlin, it gets lost in whatever “revolutionary” bullshit the Sam Altmans of the world are peddling around what is still fairly limited tech.
Look at this nonsense that I’m dealing with in my master’s finance program (sorry if you’re reading this professors, but you already know everyone is using ChatGPT anyway, this is an example you can show them of what a bad idea that is!)
A Financial Statement Effects Template (FSET) is a simple and very common method to track accounting entries. This is a basic example of what it looks like, but it might as well be quantum physics to ChatGPT.
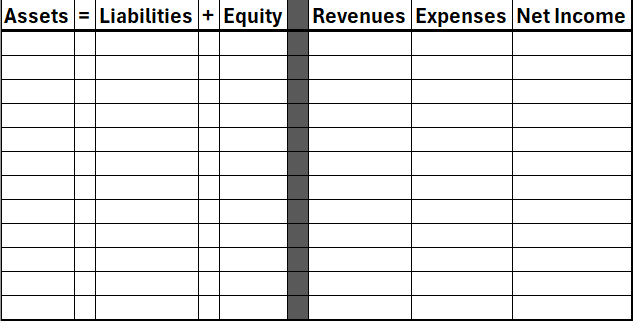
The key thing here to understand is that the cells to the right of the greyed-out area are not always utilized because not everything a business does qualifies as a revenue or expense. This is where you have to use your brain a little and where ChatGPT miserably fails.
Additionally, I included the plus and equal symbols to communicate the fundamental law of accounting, that assets must equal liabilities plus equity. If they don’t, someone screwed up somewhere. This is basic accounting logic they literally taught us on day one of class, which has clearly not been trained into ChatGPT and it simply cannot “learn” it, no matter how straightforward and simple the rules are.
ChatGPT Doesn’t Understand Any of This
If I spend $100,000 cash to buy some materials for my business, I subtract $100,000 from cash assets for the cash I spent, and add back the $100,000 to inventory under non-cash assets, because I own something now. You wouldn’t record anything on the expense or revenue side, because it is neither a revenue nor an expense. You’re just buying materials you need to generate income.
When you use the materials in your inventory to make your product, you do expense it on the income statement under cost of goods sold, and that negative net income produced by that action flows through to the equity portion of the FSET template, keeping the assets equal liabilities plus equity accounting equation in balance.
There. I just taught you a day one accounting class. Congratulations, you know more than this doofus does. I asked ChatGPT the following question:
Show me these entries on the FSET template:
1. I buy $100,000 worth of materials with cash.
2a. I use $20,000 worth of materials in making my product.
2b. I make $40,000 in revenue from those materials.
3. I take out a $10,000,000 loan to buy a new plant.
It got 1 and 3 right, demonstrating a level of understanding of the ironclad accounting rule, but the trap was set in 2 and it proved that it actually doesn’t “know” assets must equal liabilities plus equity. Here’s what ChatGPT responded with (wrong answers bolded).
2a. Transaction: Use of Materials in Production
– Impact on Assets:
– Decrease in Materials Inventory: $20,000
– Impact on Liabilities: None
– Impact on Equity: None
– Impact on Revenue: None
– Impact on Expenses: None
2b. Transaction: Revenue from Product Sales
– Impact on Assets: None
– Impact on Liabilities: None
– Impact on Equity: None
– Impact on Revenue: $40,000
– Impact on Expenses: None
When I used the word revenue, it knew to book that transaction as a revenue, but it didn’t know that money goes into your cash assets, and then it also impacts net income, which impacts equity, and it left the accounting equation unbalanced, violating the one rule you absolutely cannot break. I intentionally did not use the word “expense” in 2a, and it did not know that using materials in making a product is an expense.
When you use materials central to your revenue, that’s filed under cost of goods sold, and it’s on the top of every income statement ever. “The cost of doing business” is basically an accounting term that has achieved mass cultural adoption across the entire English-speaking world. It’s not a huge ask for these machines that are supposedly going to revolutionize the whole planet to understand the basic mechanisms of how we organize it.
But it doesn’t know there’s a monkey, or that materials used in production have multiple accounting impacts. Only whatever words it can match to its database.
Now, if I tell it to “expense” $20,000 worth of materials, ChatGPT will make the obvious fix, but still royally screw this whole thing up (wrong answers bolded).
2a. Transaction: Expense of Materials in Production
– Impact on Assets: None
– Impact on Liabilities: None
– Impact on Equity: None
– Impact on Revenue: None
– Impact on Expenses:
– Increase in Cost of Goods Sold: $20,000
This time it didn’t decrease $20,000 from assets, and just tossed $20k into cost of goods sold and called it a day while leaving expenses blank for whatever reason.
Large-language models like ChatGPT have no judgement. They “know” absolutely nothing. They are simply just the algorithmic output of an enormous database that has yielded exactly zero mass consumer products so far from these hilariously over-valued companies. Bloomberg‘s Matt Levine famously called OpenAI an $86 billion nonprofit, which is the best description of these businesses in their present state that I have seen.
ChatGPT is just fancy Google, and it’s just as bad but in different and more maddening ways. At least Google was ruined by the heedless march of capitalism and has met a familiar demise where ads have destroyed its core search product, but this stuff is just stupid on a basic level, and it offends me as a mammal with a functioning brain.
And don’t even get me started on how it’s a computer that would lose a math contest to a 3rd grader.
There are some things ChatGPT and these other large-language models are useful for. If you give it usable code, it can do a pretty good job explaining it and then building off of the rules you’ve created and save you a lot of time on repeatable tasks. My quantitative methods professor actually encouraged us to use ChatGPT sometimes to help us learn and it worked.
I can code in R now and it’s pretty fun. I would not be as advanced as I am without these LLMs shepherding me along as I wrote my code. But if you don’t have any code to begin with and ask it to write some from scratch?
Oh boy.
That’s how you make a tragicomedy.
Logic and reason are simply not a feature of LLMs because that’s just not how they “think.” So journalists who cover this stuff, please, I beg you. We need to come up with a different word for large-language models other than intelligent, because it’s an insult to all of us who can intuit what that word actually means.
GET SPLINTER RIGHT IN YOUR INBOX
The Truth Hurts